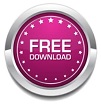

For more information about stacks, see Walkthrough: Updating a stack. These resources include an S3 bucket, Amazon SageMaker instance, and the necessary AWS Identity and Access Management (IAM) roles. Send the extracted data to the Amazon Comprehend custom model for entity extractionįor this post, we use an AWS CloudFormation stack to deploy the solution and create the resources it needs.Send the document to Amazon Textract for data extraction.Train custom entity recognition using Amazon Comprehend with the labeled data.Label the resulting data using Amazon SageMaker Ground Truth.Extract text from PDF documents using Amazon Textract.The function calls the Amazon Textract DetectDocumentText API to extract the text and calls Amazon Comprehend with the extracted text to detect custom entities. As documents are uploaded to an Amazon Simple Storage Service (Amazon S3) bucket, it triggers an AWS Lambda function. The following diagram shows a serverless architecture that processes incoming documents for custom entity extraction using Amazon Textract and custom model trained using Amazon Comprehend. The following screenshot shows the corresponding output generated using Amazon Textract and Amazon Comprehend. The following screenshot shows a sample input document. We use Amazon Textract to extract text from these resumes and Amazon Comprehend custom entity recognition to detect skills such as AWS, C, and C++ as custom entities. Use case overviewįor this post, we process resume documents from the Resume Entities for NER dataset to get insights such as candidates’ skills by automating this workflow. In this post, we show how to extract custom entities from scanned documents using Amazon Textract and Amazon Comprehend. This allows you to extract business-specific entities to address your needs. With custom entity recognition, you can to identify new entity types not supported as one of the preset generic entity types. Amazon Comprehend is a natural language processing (NLP) service that can extract key phrases, places, names, organizations, events, sentiment from unstructured text, and more. A contract document, for example, can have paragraphs of text where names and other contract terms are listed in the paragraph of text instead of as a key/value or form structure. When your organization processes a variety of documents, you sometimes need to extract entities from unstructured text in the documents. Healthcare organizations can extract patient information from documents to fulfill medical claims. For example, talent management companies can use Amazon Textract to automate the process of extracting a candidate’s skill set. This allows you to use Amazon Textract to instantly “read” virtually any type of document and accurately extract text and data without needing any manual effort or custom code.Īmazon Textract has multiple applications in a variety of fields. Textract goes beyond simple optical character recognition (OCR) to identify the contents of fields in forms and information stored in tables. Amazon Textract is a machine learning (ML) service that makes it easy to extract text and data from scanned documents.
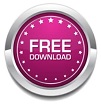